Using historical data to improve manufacturing forecasts
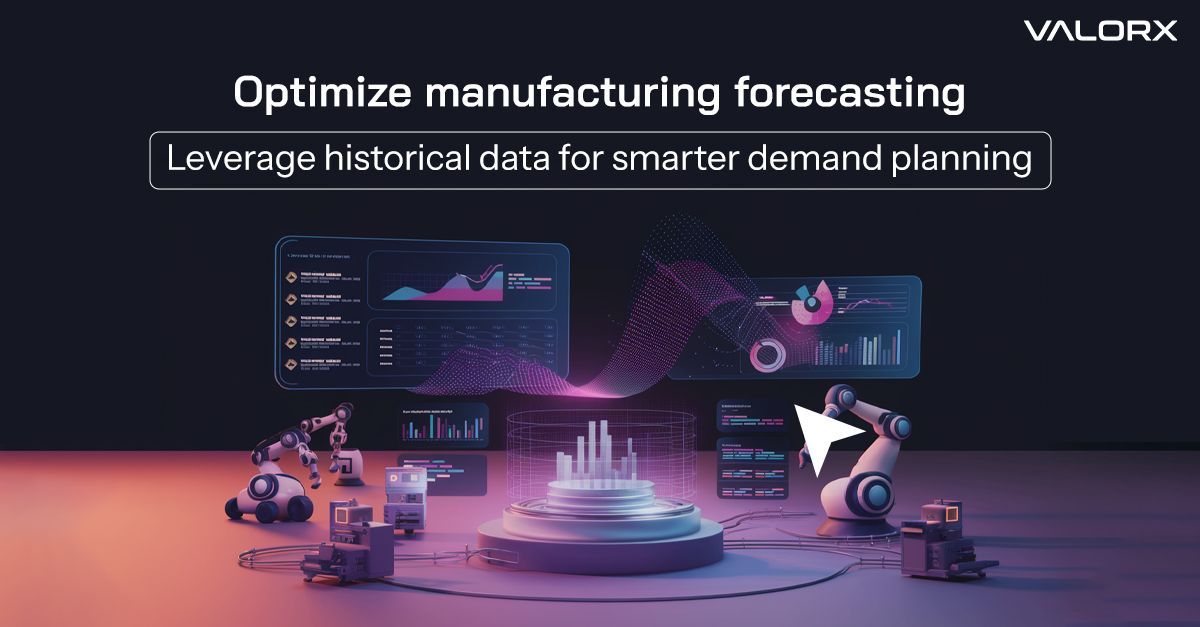
Poor forecasting has major financial implications. According to Gartner, businesses lose an estimated $12.9 million annually due to poor data quality—a key contributor to inaccurate forecasting. Overestimating demand leads to excess inventory and higher carrying costs, while underestimating it results in stockouts, lost revenue, and dissatisfied customers.
Accurate forecasting starts with clean historical data. By integrating AI-driven predictive analysis, refining data governance, and fostering cross-functional collaboration, manufacturers can turn past data into a strategic asset.
AI-driven forecasting can cut supply chain errors by 20–50%, boosting efficiency and profitability. The right data-driven approach ensures resilience and smarter decision-making in an unpredictable market.
Why historical data matters in manufacturing forecasting
Historical data forms the foundation of forecasting models, which use past trends and statistical methods to predict future demand, production needs, and market fluctuations. By analyzing past sales, production cycles, and supply chain performance, manufacturers can:
- Identify seasonal trends: Recurring demand patterns help manufacturers anticipate peak seasons and adjust inventory accordingly.
- Improve demand planning: Insights from past performance help align production schedules with expected demand, reducing waste and stockouts.
- Enhance supplier coordination: Consistent data analysis supports better planning with suppliers, ensuring raw materials are available when needed.
- Optimize workforce allocation: Predicting production cycles allows manufacturers to align labor resources with demand fluctuations.
Best practices for using historical data in forecasting
To make historical data a competitive advantage, manufacturers should integrate AI-driven analytics, refine data governance, and foster cross-functional collaboration. Key best practices include:
- Use real-time data – Combine historical trends with live sales, supplier updates, and market conditions for more accurate forecasts.
- Leverage AI and analytics – AI detects patterns, anomalies, and emerging trends that traditional methods miss.
- Segment data – Break down forecasts by product, region, or customer type for better precision.
- Continuously adjust forecasts – Regular updates and variance analysis improve responsiveness to market shifts.
- Incorporate cross-functional insights – Sales, market research, and economic data add crucial forecasting context.
By applying these strategies, manufacturers can transform historical data into actionable, high-accuracy forecasts.
Pitfalls to avoid in historical data-driven forecasting
Historical data is a powerful forecasting input that helps manufacturers make informed decisions based on past trends. However, there are common pitfalls manufacturers must avoid to ensure accurate and adaptive forecasting:
- Relying too much on past trends – Market shifts make historical data unreliable without factoring in real-time variables.
- Ignoring data quality – Incomplete or inaccurate data skews forecasts. Regular audits and clean data practices are essential.
- Overlooking external disruptions – Black swan events can derail forecasts. Factor in risk assessments to stay prepared.
- Using a one-size-fits-all approach – Different products and markets require tailored forecasting models for accuracy.
Adapting to these pitfalls ensures more precise, resilient forecasting.
The future of manufacturing forecasting
As manufacturers navigate an increasingly complex market, choosing the right forecasting approach is critical. Traditional forecasting relies heavily on historical trends, while AI-driven analytics incorporate historical data with real-time data and predictive modeling for greater accuracy.
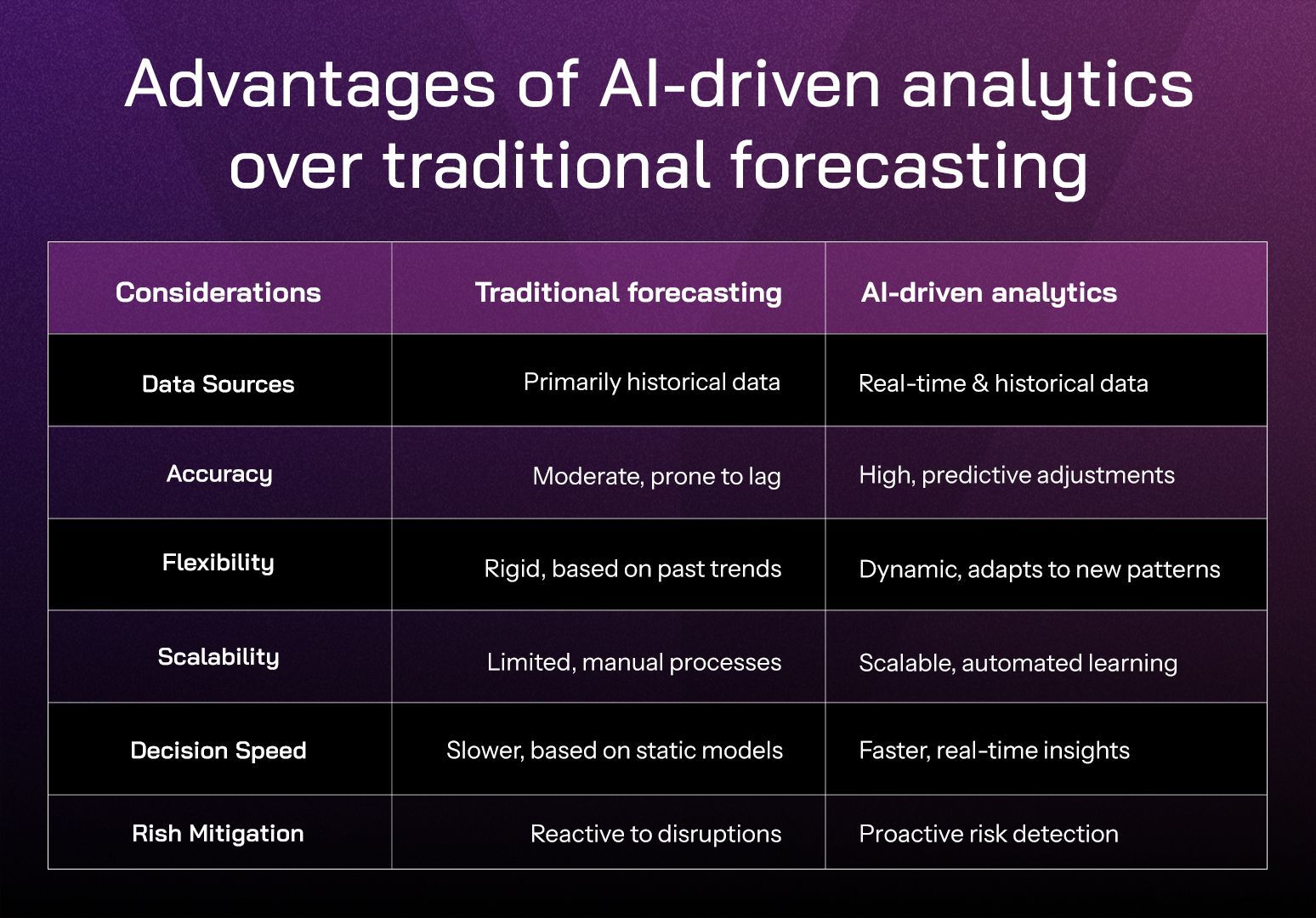
Enhancing forecasting with Valorx
Valorx Fusion provides manufacturers with an advanced forecasting solution by integrating real-time data directly into familiar spreadsheet environments. By bridging the gap between Salesforce and Excel, Valorx enables seamless data synchronization, letting users leverage historical and live data for precise forecasting.
With its bulk data editing, AI-driven analytics, and intuitive spreadsheet interface, Valorx enhances forecasting accuracy and efficiency. Manufacturers can quickly analyze trends, identify anomalies, and adjust forecasts without switching between multiple systems. Companies using Valorx report reduced forecasting errors, improved inventory management, and accelerated decision-making, ensuring they stay ahead in a dynamic market.
To see Valorx in action, watch this video showcasing how it seamlessly integrates real-time data and historical insights to enhance forecasting accuracy and decision-making.
To stay competitive in an evolving market, manufacturers must complement historical insights with modern forecasting technologies, ensuring agility, accuracy, and data-driven decision-making.
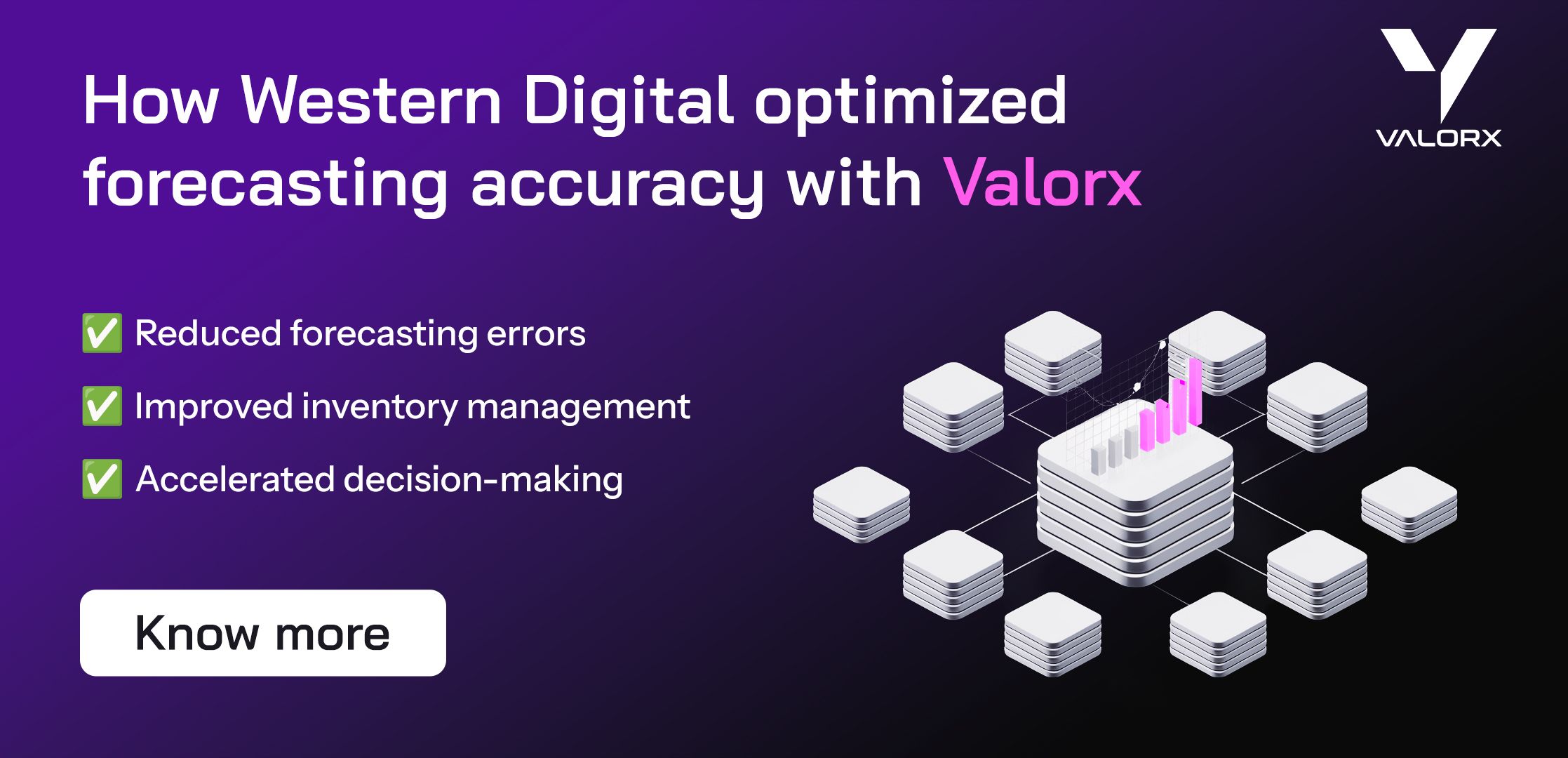
By adopting best practices and avoiding common pitfalls, manufacturers can ensure that historical data serves as a valuable asset—enhancing decision-making rather than limiting agility.